Khoury duo win pair of outstanding paper awards for AI safety research
Author: Juliana George
Date: 02.03.25
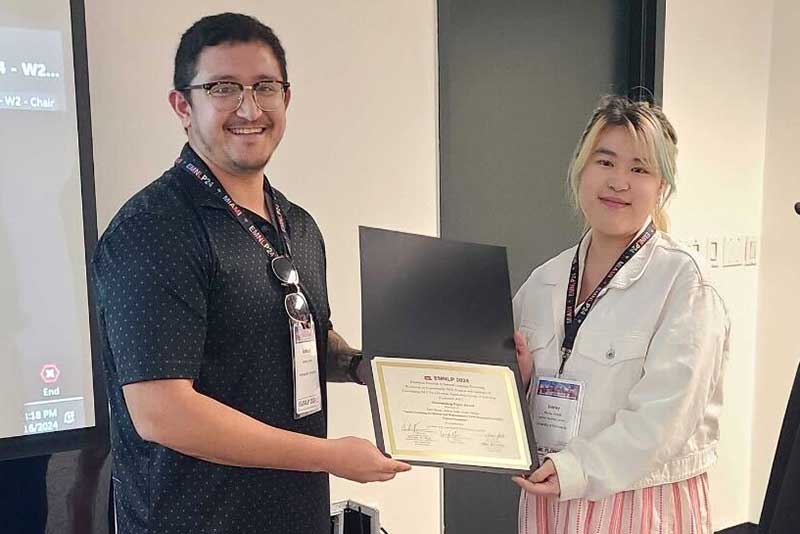
Khoury College doctoral student Anthony Sicilia knows firsthand how quickly large language models (LLMs) and conversational AI are advancing. While he sees this as positive, he also believes it’s important that AI safety protocols progress at the same rate.
Alongside his advisor, assistant professor Malihe Alikhani, Sicilia focuses on addressing uncertainty and eliminating bias in conversational AI. Similarly, Alikhani’s work emphasizes increasing accessibility and inclusivity in AI language models by integrating social science and cognitive science with machine learning.
In November, the pair, along with their coauthor Sabit Hassan, received two outstanding paper awards from workshops at the 2024 Conference on Empirical Methods in Natural Language Processing (EMNLP) in Miami. The papers focus on how LLMs can lead to safer user experiences when deployed in online web forums and other real-world environments.
“AI safety needs to be a central focus of research, especially as conversational AI is getting so good and more and more people are interacting with it,” Sicilia said. “A specific focus of AI safety in these papers is how user behaviors can be influenced by large language models.”
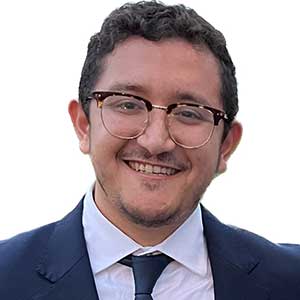
For the first paper, “Eliciting Uncertainty in Chain-of-Thought to Mitigate Bias against Forecasting Harmful User Behaviors,” the researchers used Reddit conversations to test the accuracy of five LLMs in predicting personal attacks and other harmful user behavior on social media. They found that while the models tended to have a bias against predicting harmful events, this bias could be reduced by asking the model to rate the likelihood of a personal attack on a 10-point scale based on a conversation fragment, and by asking it to explain its reasoning in a chain-of-thought prompt such as “let’s think step by step.”
Sicilia and Alikhani believe that their findings could help make social media a safer place, ensuring that potentially hostile interactions don’t slip through the cracks of existing moderation systems.
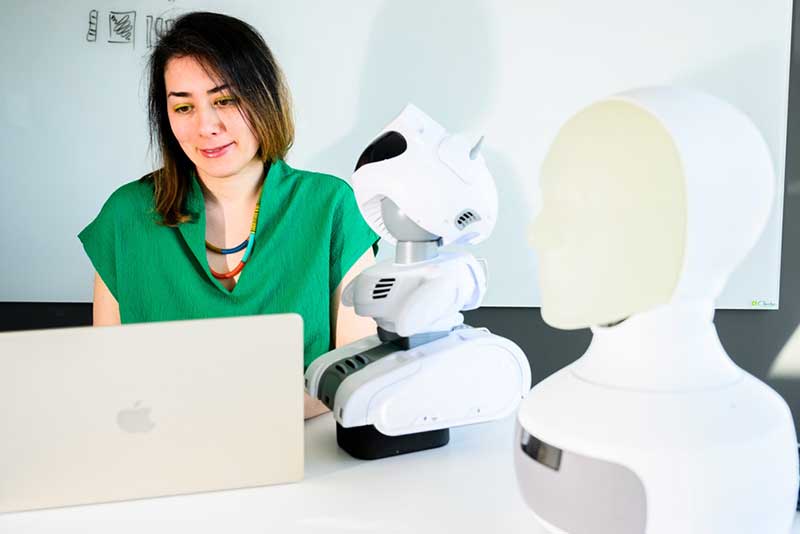
“The findings demonstrate how asking language models to represent their uncertainty can reduce biases and improve accuracy, especially when working with limited data,” Alikhani said. “This is particularly important for applications like social media moderation, where biases can have real-world consequences.”
The second paper, “Active Learning for Robust and Representative LLM Generation in Safety-Critical Scenarios,” stemmed from a project Sicilia and Alikhani worked on together last year for Amazon’s Alexa Prize TaskBot Challenge, in which their team placed third. The competition required participants to create a task-oriented AI assistant or “TaskBot” that interacted with real Alexa users. To refine the TaskBot’s safety system, the team created an LLM-generated simulated data set with 5,400 potential safety violations using active learning and clustering. This process trained the model to anticipate safety concerns for users in a wide range of situations based on their dialogue.
READ: Khoury inclusive AI team places third in Amazon’s Alexa Prize TaskBot Challenge
“Whenever the safety system senses the user is talking about something that it should not respond to, or that is unsafe and might require a 911 call, then it would trigger some template response that says, ‘This is out of my jurisdiction. You need to seek out help,’ or something like that,” Sicilia explained.
Potential safety violations ranged from health emergencies to complex legal problems to self-harm, none of which language models are equipped to handle. Despite the relative rareness of these situations, Sicilia and Alikhani believe that it’s important for AI training to factor in the possibility of unexpected safety-critical scenarios. What’s more, Sicilia noted that the data set the team created for the paper could be useful for training other safety systems, so they’ve made it publicly available.
The team’s hard work culminated in their presenting of their papers at EMNLP, where both earned outstanding paper awards.
“I wasn’t necessarily surprised, I thought they were pretty strong works,” Sicilia said of the win. “But it’s nice to receive the recognition, and I think it’s important work.”
Sicilia is in his final year as a doctoral student and hopes to start his own lab, which will continue research to improve the communication capabilities of conversational AI models. Likewise, Alikhani was overjoyed at the success of both papers and wants to continue pursuing projects that advance AI accessibility, in line with Khoury College’s core mission of “computer science for everyone.”
“AI should benefit everyone, not just a select few,” she said. “This means creating technologies that are inclusive and representative, with a particular emphasis on safety-critical applications.”
The Khoury Network: Be in the know
Subscribe now to our monthly newsletter for the latest stories and achievements of our students and faculty