Khoury News
The unusual challenges and neurological benefits of tracking toddlers with machine learning
By using multiple cameras and machine learning algorithms to track toddlers, an interdisciplinary Northeastern research team is striving to identify neurological disorders and intervene earlier.
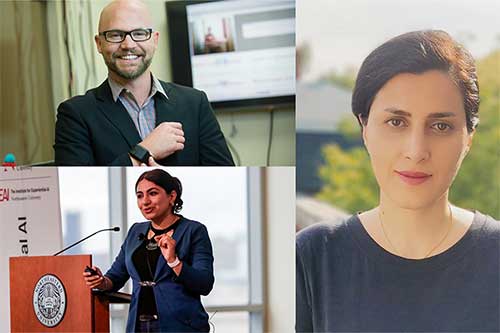
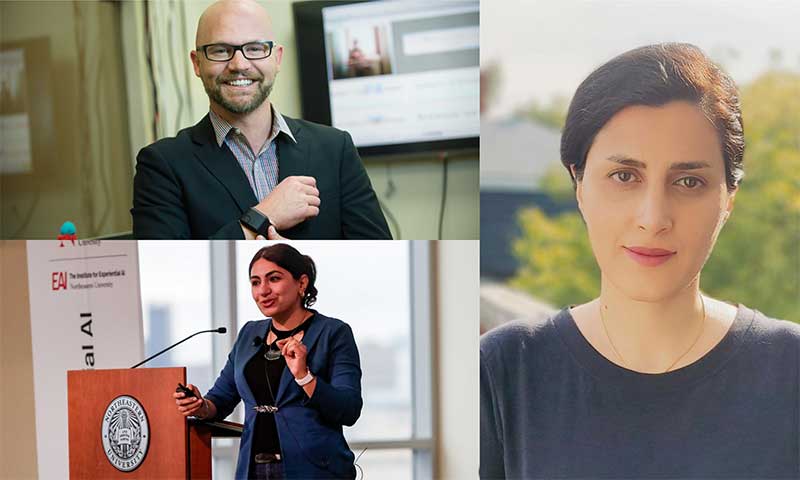
The ongoing boom in tracking technology has enabled innovation in the security realm, such as facial recognition systems used by organizations like the TSA. Lesser known, however, is how the technology is used to track toddlers.
Toddlers' unpredictable movements and smaller number of defining features compared with adults have made them difficult to track. But a team of Northeastern researchers has recently taken steps to overcome these challenges, using multicamera tracking and new machine learning algorithms to better recognize and differentiate toddlers.
While tracking toddlers might sound a bit ominous, the technology is intended to recognize features associated with neurological disorders that would be difficult for people to identify on their own. Early detection of these disorders can allow for more timely intervention, which can greatly reduce how much a given disorder might impact someone’s life as they get older.
The project is a collaboration between Matthew Goodwin, a professor jointly appointed in Khoury College and the Bouvé College of Health Sciences, and Sarah Ostadabbas, a professor in the College of Engineering (CoE). The pair met through Northeastern’s Institute for Experiential AI (EAI), where they resolved to hire a postdoctoral researcher to assist them with automated detection of human behavior, especially as it relates to motor development and behavior of autistic individuals.
That postdoc was Somaieh Amraee, who studies at CoE and is also a part of the EAI. She collaborated with Ostadabbas at the Augmented Cognition Lab — which Ostadabbas directs — and had a multiperson and multicamera tracking background that made her perfect for the project.
Along with Amraee, the team recruited Bishoy Galoaa, an electrical and computer engineering master's student who is being mentored by Amraee.
“He was instrumental in creating the multicamera multiperson tracking algorithms in my lab,” Ostadabbas said.
The team believes that tracking technologies could help detect early signs of autism spectrum disorder (ASD) in toddlers, which would be incredibly beneficial for parents and other caretakers of young children.
“If you have an eight-hour video [of toddlers], imagine how time-consuming it would be to watch the entire footage,” Ostadabbas said. “Our goal is to develop computer vision technology that can automatically summarize such videos, extracting the most relevant moments. Additionally, we aim to create systems capable of real-time alerts, notifying parents or daycare personnel if specific critical actions or behaviors occur, ensuring a safer and more efficient childcare environment.”
Many indicators of neurological disorders in young children can be seen in their movements.
“In many pediatric disorders, some of the earliest features that cause concern about failing to meet development milestones … are impairments or differences in motor movement,” Goodwin said. “How are they proceeding from laying down to sitting up to crawling to walking to reaching to grasp? These are all motor signs with typical trajectories in the normative population ... we can compare to.”
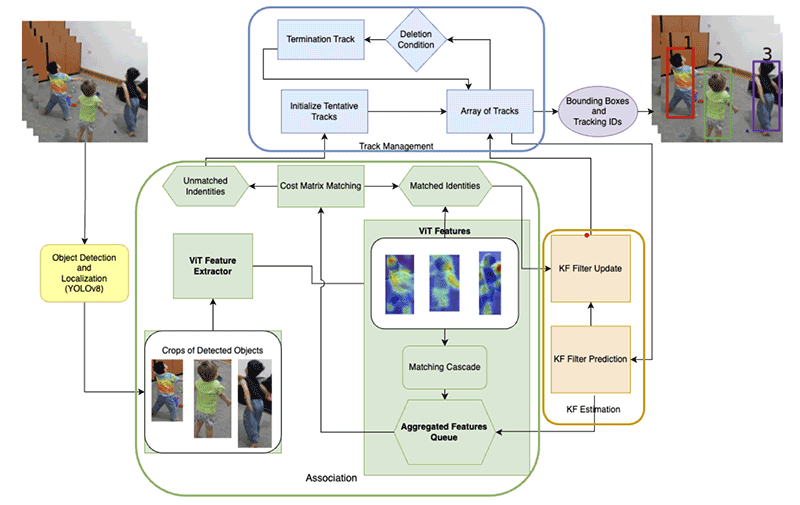
Beyond not meeting development milestones, tics and other repetitive behaviors can also serve as early signs of potentially serious and even life-threatening disorders. Detecting such behaviors early is essential to providing families with the best support to help raise their children, according to Goodwin.
“The brain is always rewiring, but we see the most change in neurological development in the first years of life,” said Goodwin. “So, if children are missing developmental milestones or are on a different trajectory early on, the sooner we can identify them and provide intervention that promotes developmental outcomes, the better. The later we wait to intervene, the less neural rewiring based on environmental support we see.”
The team believes that more automated methods of tracking toddler behavior may allow for more equitable diagnosis of these disorders, many of which, including ASD, have sharp race-and gender-based inequalities when it comes to diagnoses.
“The American Academy of Pediatrics has mandatory screening for autism at 12, 24, and 36 months, even though we don’t diagnose officially until 36 months,” Goodwin said. “The average age of diagnosis is usually three to four years old, but if you’re not white, it’s eight years old. These kids are not coming to clinics in an equitable way.”
The team began developing their toddler tracking technology by addressing the major roadblocks that previous researchers faced. First was a lack of publicly accessible video data on toddlers due to privacy and security issues. Ostadabbas had encountered this challenge on a previous project involving infants and computer vision, an endeavor that eventually won her the National Science Foundation CAREER award with Goodwin as a mentor.
“We learned from that study you could [pull extra video data] from social media and use it to test your model,” Ostadabbas said.
The team also added data by working with the Marcus Autism Center, a Georgia-based clinic and close collaborator of Goodwin’s that specializes in intervention and evaluation for children with ASD.
After getting the data they needed, the team had to address another serious challenge: developing successful multicamera tracking. This method would allow for a more comprehensive view of each toddler, but with the increased number of cameras came increased potential for complications. Many multicamera tracking technologies work by “using the average” of the information they receive from each camera. While this sounds practical in theory, in reality these averages are often muddied by major outliers such as an item blocking one camera's view.
“We realized that adding an extra camera doesn’t just provide more information. It also introduces more challenges that we have to be mindful of,” Ostadabbas said. “It requires using them intelligently to make sure the result is robust and less error is introduced to the tracking problem.”
To address the issue, the researchers created an algorithm that presented a more representative view of the data. They developed a genetic algorithm that mimics the process of natural selection to continuously optimize the outputs of an algorithm until more and more accurate results are reached. And they added two data modules, one focused on movement and another on feature recognition, to help the algorithm differentiate toddlers and avoid confusion based on their unpredictable movements.
Their success represents a step forward in toddler tracking technology, particularly within preventive health care. Even beyond the tracking technology itself, much of the data used for the project will likely have lasting impacts on the field.
“[The team] is creating data sets that families have given them permission to share with other investigators,” Goodwin said. “That’s going to help other people who are developing tracking methods; they can work with the same data and compare and contrast their performance with the performance presented in our paper.”
Olivia Mintz contributed reporting to this story.
The Khoury Network: Be in the know
Subscribe now to our monthly newsletter for the latest stories and achievements of our students and faculty